Is Your Data & Analytics Ecosystem Still Stuck in 2019?
Welcome to our Inaugural Blog Post!
While everyone is talking about AI transformation, a (not) shocking 78% of enterprises are still struggling with basic data quality issues, according to a recent report from McKinsey. At a time when AI capabilities are advancing at breakneck speed, most organizations remain trapped by fundamental data challenges that prevent them from truly harnessing the power of artificial intelligence.
The Hidden Reality Behind AI Transformation Failures
The AI revolution promises unprecedented business value, but there's a big disconnect between ambition and execution. Despite significant investments in AI technology, many organizations find themselves unable to generate meaningful ROI, or even deploy useful solutions into their environments to begin with. The culprit? Well, there are lots, as it turns out. We’ll get to those in future articles... For the sake of brevity today, we’ll focus on neglected data foundations.
Consider these realities:
- Companies with robust data infrastructures are 3x more likely to report successful AI implementations
- Only 22% of enterprises have data ecosystems mature enough to support advanced AI applications
- Poor data quality costs businesses an average of $12.9 million annually (according to Gartner). This absolutely does not take into account the jaw dropping economics companies can achieve through AI.... Watch this metric grow exponentially...
Breaking the Data Quality Barrier
The path to AI transformation doesn't begin with algorithm selection or model deployment—it starts with establishing data excellence across your entire ecosystem. This means:
Data Governance That Powers Innovation
Modern data governance isn't about restriction—it's about enabling speed and confidence, it’s about democratization. By implementing intelligent governance frameworks, organizations can simultaneously protect sensitive information while accelerating access to high-quality data assets.
Integration Without Complexity
Data silos remain the most persistent obstacle to AI readiness. (Although our thinking on this is actually evolving as we speak...) New integration approaches can unify disparate data sources without creating brittle architectures that break with every system update. In fact, our professional opinion is that smart AI tooling can be a major shortcut in this department...
Quality as a Continuous Process
Data quality isn't achieved through one-time cleansing efforts. Leading organizations are implementing automated quality monitoring that identifies and resolves issues before they impact business operations.
The Evolution Imperative
Organizations that fail to evolve their data foundations will increasingly fall behind competitors who can rapidly deploy and scale AI solutions. The gap between data leaders and laggards will only widen as AI capabilities continue advancing. Seriously, the disruption in this space is coming faster than most of us fully appreciate.
How We're Changing the Equation
At Enlighten, our approach bridges the gap between data reality and AI aspiration by focusing on four critical dimensions:
1/ Accelerated Data Maturity: We compress the typical data evolution timeline from years to months through our proprietary assessment and transformation methodology. How? We do NOT take a platforms-up approach advocating for massive data engineering and transformation programs. Instead, we focus on the INSIGHTS that actually drive business value, the levers of power, and KPIs that drive max value and work backwards from there.
2/ Intelligent Automation: Our solutions apply AI to solve data quality problems, creating a virtuous cycle where better data enables better AI.
3/ Business-Centric Focus: We align all data quality improvements directly to measurable business outcomes and use cases, ensuring ROI from day one.
4/ Data Governance: Governance is inherently a text, communication, and compliance problem. AI is extremely well suited to address this problem. Tools like Collibra are excellent platforms for managing data standards and definitions, but lack processes to sync data understanding across teams and AI data agents. AI can be a tool which closes the gap between data standards, definitions, and usage parameters in master data tools, and the practical consumption and orchestration requirements from Analysts. This shared understanding is critical to enabling AI data agents to DO anything of any practical use.
Take the First Step Toward True AI Transformation
Don't let poor data quality hold your AI initiatives hostage. The organizations that will thrive in the AI era are taking decisive action today to evolve their data ecosystems.
Contact us to discover how our solutions can transform your data foundation from an obstacle into your greatest competitive advantage. Together, we can ensure your organization isn't left behind in the AI revolution.
#AITransformation #DataEvolution #DataQuality #EnterpriseAI #DigitalTransformation
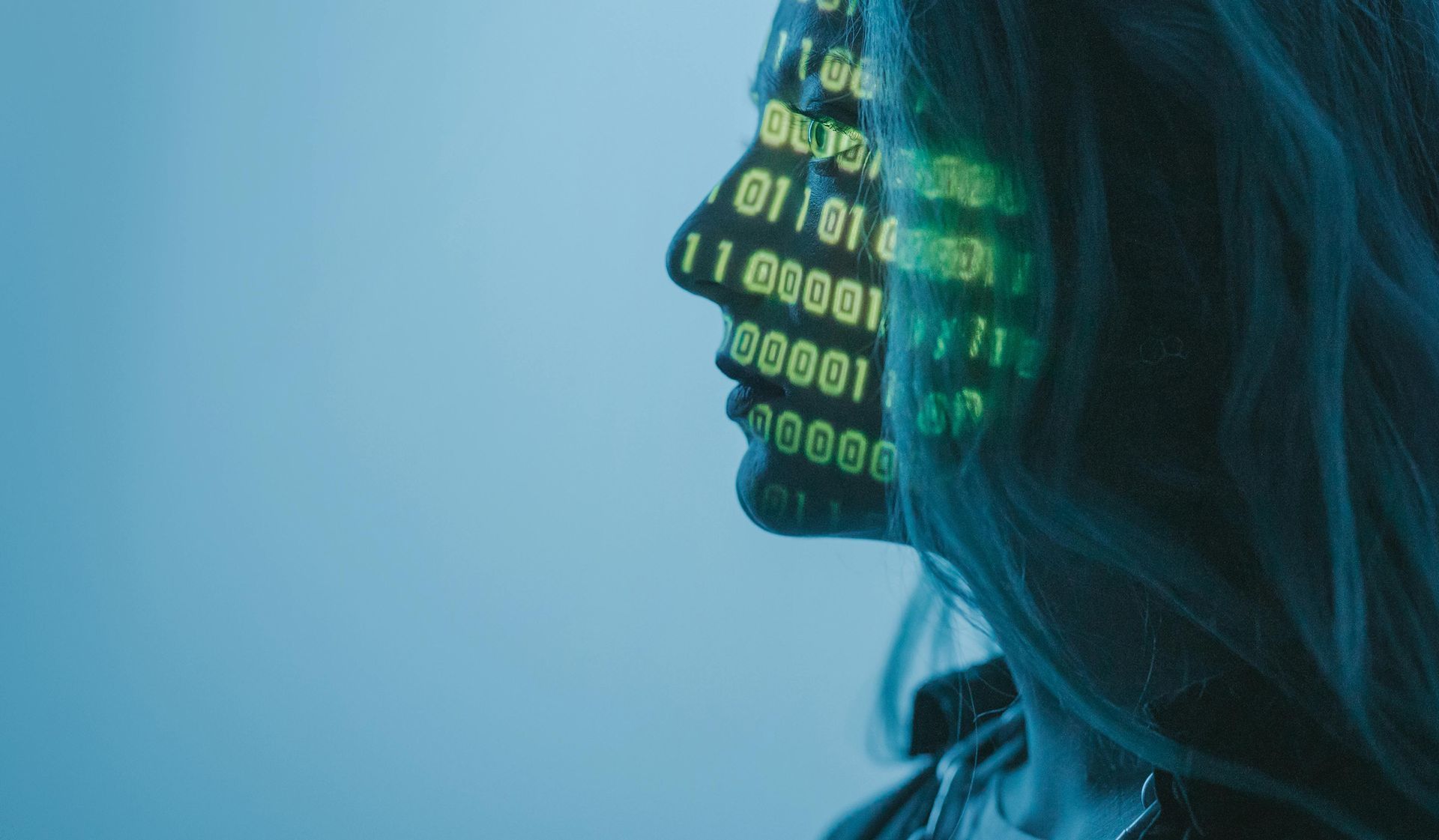