Hot Take: Most AI Implementations Are Just Expensive, High-Risk Experiments in Disguise
Let's be brutally honest: the AI landscape is littered with expensive proof-of-concepts that never deliver real ROI or get anywhere close to production. Companies are rushing to implement AI solutions that look impressive in demos but collapse when faced with actual business complexity. Look, we can all appreciate that everyone MUST have a point of view and a strategy around AI these days, but the trend of slapping a chatbot on some data and calling it a day is quickly running out of steam. The culprit? A fundamental misunderstanding of what makes AI truly valuable.
The Chatbot Delusion
We've all seen it: the slick chatbot demo that wows executives but falls apart when deployed. These AI vanity projects consume millions in investment while delivering minimal business impact. They represent the worst kind of digital theater – impressive on the surface but hollow underneath. Worse yet, many early pilots have actually yielded WORSE customer outcomes and broad frustration.
The Multi-Faceted Challenge of Real AI Success
While organizations race to deploy generative AI applications, they're ignoring several crucial realities that determine success or failure:
Context Is King, But Painfully Elusive
AI models may be trained on vast swaths of internet data, but they remain fundamentally clueless about your specific business context. The specialized knowledge of your industry, company processes, and unique market position – the elements that actually drive competitive advantage – are precisely what generic AI models lack.
Teaching AI to understand these contextual nuances often proves far more challenging than companies anticipate. It's not just about feeding documents; it's about encoding complex institutional knowledge and decision frameworks that have evolved over years and decades.
The Integration Nightmare
Even technically successful AI projects often fail at the crucial moment of integration into existing workflows. The most powerful AI capabilities are worthless if they create friction in daily operations. Users quickly abandon tools that feel bolted-on rather than naturally embedded in their work processes.
Organizations underestimate the design challenge of delivering AI insights at exactly the right moment, in exactly the right format, without disrupting established patterns of work.
The Hallucination Problem
AI systems confidently presenting false information as fact remains a persistent challenge that undermines trust and adoption. In consumer applications, hallucinations might be amusing. In business contexts, they can be disastrous.
Companies struggle to implement effective verification mechanisms that balance the need for accuracy with the speed and autonomy that make AI valuable in the first place.
Data Realities Still Matter
While robust data platforms aren't the only prerequisite for AI success, data quality and accessibility remain crucial challenges. Organizations often discover that their data is:
- Siloed across incompatible systems
- Inconsistently formatted or labeled
- Missing critical information needed for meaningful insights
- Insufficient in volume for effective AI training
Breaking the Cycle of AI Disappointment
To avoid becoming another AI failure statistic, organizations must adopt a more nuanced, holistic approach:
- Prioritize context acquisition: Invest in systematic methods to capture and encode business context that generic models lack
- Design for workflow integration: Start with how work actually happens, then determine how AI can enhance rather than disrupt those patterns
- Implement practical trust mechanisms: Build verification processes that balance accuracy with speed and autonomy
- Take a pragmatic data approach: Focus on making specific data assets useful rather than boiling the ocean with massive platform projects
- Take a Command-Center approach to AI: AI is not an “easy button.” Decompose complex tasks into bite-sized pieces and apply AI solutioning that brings institutional knowledge, in an auditable and repeatable way, to reduce the friction of everyday work.
The Competitive Divide Is Growing
Organizations that address these multifaceted challenges are quietly outpacing competitors fixated on visible but ineffective AI applications. This performance gap will only widen as AI technologies mature.
The choice is clear: continue investing in impressive but ineffective AI showcases, or build AI systems that genuinely enhance how work gets done in your unique business context. Which side of the AI reality will your organization end up on?
#AIReality #ContextMatters #WorkflowIntegration #EnterpriseAI #DigitalTransformation
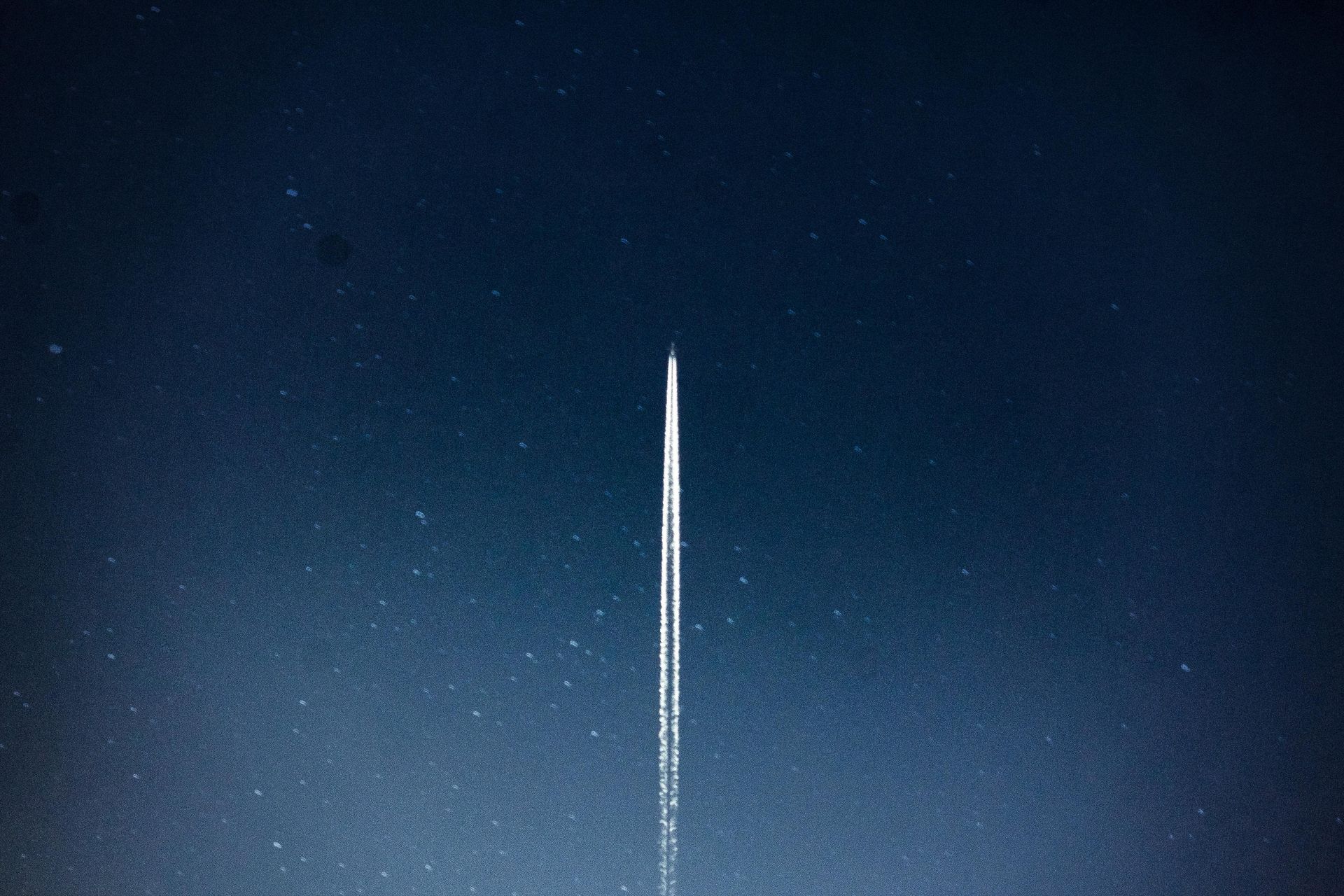